#260: Hyundai’s Exoskeletons, with Sangin Park
In this interview, Audrow Nash speaks with Sangin Park, Senior Research Engineer at Hyundai, about exoskeletons. Park describes three exoskeleton prototypes: one for helping workers reduce back pain, one for assisting a person with paraplegia, and an exoskeleton for soldiers. Park discusses the sensors and actuators of each exoskeleton, as well as Hyundai’s exoskeleton ambitions.
Sangin Park
Sangin Park is a Senior Research Engineer at Hyundai.
Links
Walking robots, a YouTube sensation, get ready for market
Humanizing Exoskeletons for Soldiers, Workers and Paraplegics
Stepping It Up A Notch
Robot transitions from soft to rigid
By Leah Burrows
Even octopuses understand the importance of elbows. When these squishy, loose-limbed cephalopods need to make a precise movement — such as guiding food into their mouth — the muscles in their tentacles contract to create a temporary revolute joint. These joints limit the wobbliness of the arm, enabling more controlled movements.
Now, researchers from the Wyss Institute for Biologically Inspired Engineering at Harvard University and the Harvard John A. Paulson School of Engineering and Applied Sciences (SEAS) have shown how a multi-layered structure can allow robots to mimic the octopus’ kinematics, creating and eliminating joints on command. The structure can also allow robots to rapidly change their stiffness, damping, and dynamics.
The research is published in two papers in Advanced Functional Materials and IEEE Robotics and Automation Letters.
“This research helps bridge the gap between soft robotics and traditional rigid robotics,” said Yashraj Narang, first author of both studies and graduate student at SEAS. “We believe that this class of technology may foster a new generation of machines and structures that cannot simply be classified as soft or rigid.”
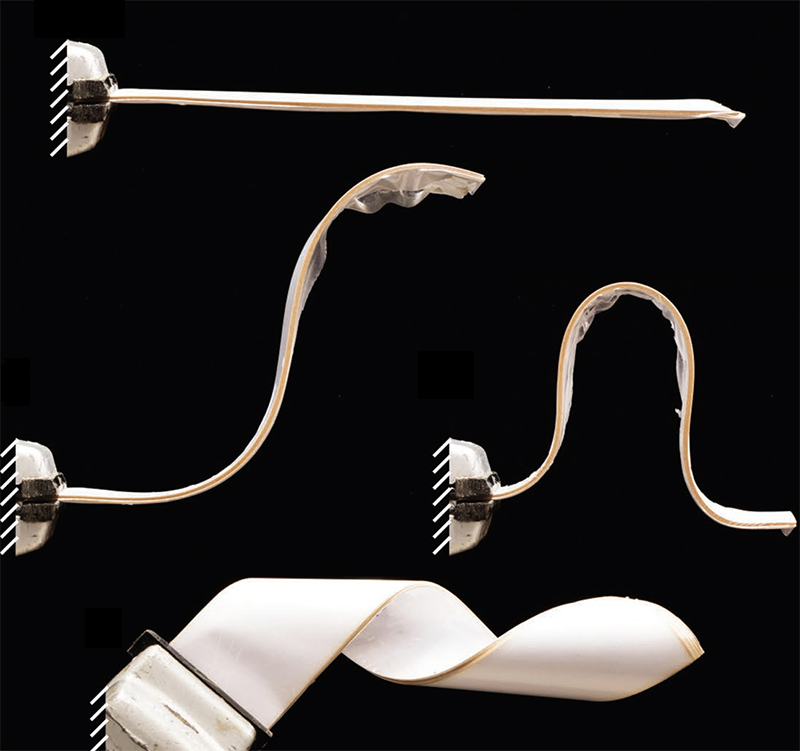
The structure is surprisingly simple, consisting of multiple layers of flexible material wrapped in a plastic envelope and connected to a vacuum source. When the vacuum is off, the structure behaves exactly as you would expect, bending, twisting and flopping without holding shape. But when a vacuum is applied, it becomes stiff and can hold arbitrary shapes, and it can be molded into additional forms.
This transition is the result of a phenomenon called laminar jamming, in which the application of pressure creates friction that strongly couples a group of flexible materials.
“The frictional forces generated by the pressure act like glue,” said Narang. “We can control the stiffness, damping, kinematics, and dynamics of the structure by changing the number of layers, tuning the pressure applied to it, and adjusting the spacing between multiple stacks of layers.”
The research team, which included Wyss Associate Faculty member Robert Howe, Ph.D., the Abbott and James Lawrence Professor of Engineering at SEAS; Joost Vlassak, Ph.D., the Abbott and James Lawrence Professor of Materials Engineering at SEAS; and Alperen Degirmenci, a SEAS graduate student, extensively modeled the mechanical behavior of laminar jamming to better control its capabilities.
Next, they built real-world devices using the structures, including a two-fingered gripper that, without a vacuum, could wrap around and hold onto large objects and, with a vacuum, could pinch and hold onto small objects about the size of a marble.
The researchers also demonstrated the structure’s capabilities as shock absorbers by attaching them to a drone as a landing gear. The team tuned the stiffness and damping of the structures to absorb the impact of landing.
The structure is a proof-of-concept that could have many applications in the future, from surgical robots to wearable devices and flexible speakers.
“Our work has explained the phenomenon of laminar jamming and shown how it can provide robots with highly versatile mechanical behavior,” said Howe, who is the senior author of the paper. “We believe that this technology will eventually lead to robots that can change state between soft, continuous devices that can safely interact with humans, and rigid, discrete devices that can meet the demands of industrial automation.”
This research was supported in part by the National Science Foundation.
Insight into a development process: The robotic fabrication of concrete facade mullions with smart dynamic casting
After the successful completion of the production of the material-optimised concrete façade mullions, Fabio Scotto and Ena Lloret-Frischti of the Gramazio Kohler Research Group at ETH Zurich and the Chair for Physical Chemistry of Building Materials, ETH Zurich take a look back at the experiments and prototypes which were necessary in the development of a final robotic fabrication process.
The integration of Smart Dynamic Casting (SDC) for the production of the façade mullions for the first floor of DFAB HOUSE has led us to the development of an adaptive robotic setup which allows us to produce custom-made reinforced concrete structures. Until the final development of a robust robotic process, we had to overcome several challenges during the experimental and prototypical phase.
Scaling down the production system and minimizing the friction forces
Our first main task was to scale down the production system to realise structures with a minimal cross section of 100×70 mm. This had a direct impact on the formwork system we were working with previously. We observed an increase in friction forces that resulted in accumulations of concrete along the inside walls of the formwork. This caused major issues during the casting process, specifically surface disruptions and, in some cases, a complete flow out of the fresh concrete.
To minimize the effects of the friction forces, we have defined strategies to optimize the overall process. Firstly, we engineered a much more self-compacting concrete mix with greater flowability and optimised setting times. Secondly, the formwork material was changed to stainless steel. Together with our capillary oiling concept, this ensures a significantly more frictionless casting process.
Integration of the reinforcement
Another issue we had to overcome in the research process was the integration of the reinforcement system. Although the mullions for DFAB HOUSE are not load-bearing, their structural performance is optimized for wind and self-weight load.
We developed a non-standard reinforcement system constrained within the small cross sections of the mullions. Two 12mm radius stainless steel bars, previously bent into the desired shape through CNC processes, are welded together through horizontal connection elements. The reinforcement is placed inside the formwork prior to initiating the fabrication process and fixed to lower and upper attachments. Due to the slender geometries of the mullions, the reinforcement had the tendency of bending under the effect of its own weight and the attachment system has been reviewed to avoid any deflection during the concrete casting. We devised a pulley system that allows us to fix the reinforcement in place by applying tension. In doing so, we ensure the reinforcement is held straight throughout the whole production, minimizing deflections along the entire height of the mullion.
A successful development process
After numerous experiments and prototypes, we have successfully overcome all the challenges that arose in the process and finalised an optimised fabrication procedure. The SDC mullions produced for the DFAB HOUSE display great surface quality while fulfilling the required structural criteria.
Getting robotic surgical tools from the lab to the operating room
What’s Driving Investments in Surgical Robots?
PRE-TEC Integrates ATI Tool Changers in a Fast Finishing Solution
DJI And Microsoft Partner To Bring Advanced Drone Technology To The Enterprise
Researchers teach robots to touch
Robots in Depth with Linda Thayer
In this episode of Robots in Depth, Per Sjöborg speaks with Linda Thayer about how to apply for, maintain and use patents.
Linda tells us about the benefits of getting in touch with a patent attorney early in the innovation process. She then walks us through the process of applying for a patent, key dates and important steps.
We also get to hear about defending your patent and international patents.
Self-driving cars for country roads
A team of MIT researchers tested MapLite on a Toyota Prius outfitted with a range of LIDAR and IMU sensors.
Photo courtesy of CSAIL.
By Adam Conner-Simons | Rachel Gordon
Uber’s recent self-driving car fatality underscores the fact that the technology is still not ready for widespread adoption. The reality is that there aren’t many places where today’s self-driving cars can actually reliably drive. Companies like Google only test their fleets in major cities, where they’ve spent countless hours meticulously labeling the exact 3-D positions of lanes, curbs, and stop signs.
“The cars use these maps to know where they are and what to do in the presence of new obstacles like pedestrians and other cars,” says Daniela Rus, director of MIT’s Computer Science and Artificial Intelligence Laboratory (CSAIL). “The need for dense 3-D maps limits the places where self-driving cars can operate.”
Indeed, if you live along the millions of miles of U.S. roads that are unpaved, unlit, or unreliably marked, you’re out of luck. Such streets are often much more complicated to map, and get a lot less traffic, so companies aren’t incentivized to develop 3-D maps for them anytime soon. From California’s Mojave Desert to Vermont’s White Mountains, there are huge swaths of America that self-driving cars simply aren’t ready for.
One way around this is to create systems advanced enough to navigate without these maps. In an important first step, Rus and colleagues at CSAIL have developed MapLite, a framework that allows self-driving cars to drive on roads they’ve never been on before without 3-D maps.
MapLite combines simple GPS data that you’d find on Google Maps with a series of sensors that observe the road conditions. In tandem, these two elements allowed the team to autonomously drive on multiple unpaved country roads in Devens, Massachusetts, and reliably detect the road more than 100 feet in advance. (As part of a collaboration with the Toyota Research Institute, researchers used a Toyota Prius that they outfitted with a range of LIDAR and IMU sensors.)
“The reason this kind of ‘map-less’ approach hasn’t really been done before is because it is generally much harder to reach the same accuracy and reliability as with detailed maps,” says CSAIL graduate student Teddy Ort, who was a lead author on a related paper about the system. “A system like this that can navigate just with on-board sensors shows the potential of self-driving cars being able to actually handle roads beyond the small number that tech companies have mapped.”
The paper, which will be presented in May at the International Conference on Robotics and Automation (ICRA) in Brisbane, Australia, was co-written by Ort, Rus, and PhD graduate Liam Paull, who is now an assistant professor at the University of Montreal.
For all the progress that has been made with self-driving cars, their navigation skills still pale in comparison to humans’. Consider how you yourself get around: If you’re trying to get to a specific location, you probably plug an address into your phone and then consult it occasionally along the way, like when you approach intersections or highway exits.
However, if you were to move through the world like most self-driving cars, you’d essentially be staring at your phone the whole time you’re walking. Existing systems still rely heavily on maps, only using sensors and vision algorithms to avoid dynamic objects like pedestrians and other cars.
In contrast, MapLite uses sensors for all aspects of navigation, relying on GPS data only to obtain a rough estimate of the car’s location. The system first sets both a final destination and what researchers call a “local navigation goal,” which has to be within view of the car. Its perception sensors then generate a path to get to that point, using LIDAR to estimate the location of the road’s edges. MapLite can do this without physical road markings by making basic assumptions about how the road will be relatively more flat than the surrounding areas.
“Our minimalist approach to mapping enables autonomous driving on country roads using local appearance and semantic features such as the presence of a parking spot or a side road,” says Rus.
The team developed a system of models that are “parameterized,” which means that they describe multiple situations that are somewhat similar. For example, one model might be broad enough to determine what to do at intersections, or what to do on a specific type of road.
MapLite differs from other map-less driving approaches that rely more on machine learning by training on data from one set of roads and then being tested on other ones.
“At the end of the day we want to be able to ask the car questions like ‘how many roads are merging at this intersection?’” says Ort. “By using modeling techniques, if the system doesn’t work or is involved in an accident, we can better understand why.”
MapLite still has some limitations. For example, it isn’t yet reliable enough for mountain roads, since it doesn’t account for dramatic changes in elevation. As a next step, the team hopes to expand the variety of roads that the vehicle can handle. Ultimately they aspire to have their system reach comparable levels of performance and reliability as mapped systems but with a much wider range.
“I imagine that the self-driving cars of the future will always make some use of 3-D maps in urban areas,” says Ort. “But when called upon to take a trip off the beaten path, these vehicles will need to be as good as humans at driving on unfamiliar roads they have never seen before. We hope our work is a step in that direction.”
This project was supported, in part, by the National Science Foundation and the Toyota Research Initiative.
Warehousing, fulfillment and DC transformation trends
E-commerce sales for 2017 were $453.5 billion in the U.S. and $1.1 trillion in China, an increase of 16.0% and 32.6% respectively over 2016. This upward trend is projected to continue for the next many years. Consequently flexibility and an ability to handle an ever-increasing number of parcels is of concern to warehousing, fulfillment and distribution center (DC) managers around the world.
Handling, distribution, transport and delivery – and the amortization of facility setup charges which often represent more cost than raw materials and manufacturing combined – are part of mounting challenges faced by today’s fulfillment executives. Accordingly, warehousing and material handling are a big business for hundreds of different types of companies that provide conveyors, rollers, racks, vision systems, hoists, shelving, electric motors, slides, barcode readers, printers, ladders, gantries, tugs, forklifts, skids, totes, carts, and software systems of all types. Most of these vendors provide products which serve the man-to-goods model, ie, a person goes somewhere in the warehouse, finds the item, and either puts it into further play in the system or packs it himself.
Kiva Systems shattered that model with their goods-to-person robots and dynamic shelving systems. Amazon was so enamored with Kiva’s robotic solution that it acquired Kiva and their robots. Since that acquisition Amazon Robotics (as Kiva Systems was renamed) has since produced over 130,000 Kiva robots and put them all to work in Amazon warehouses and DCs thus proving the efficacy of the method – a method which has been copied and also expanded upon by multiple vendors listed below.Bottom line: In warehouse and supply chain logistics focused on e-commerce fulfillment, whether third-party logistics service providers or e-retailers and their logistics arms, fixed and exorbitant front-end costs for conveyors, elevators and old style AS/RS systems have become anathema to warehouse executives worldwide who are clamoring to lower fixed costs while increasing flexibility and handling more goods. Comprehensive software and analytics — particularly predictive analytics — are on executives near-term agendas. Hence the need to invest in NextGen Supply Chain methods offered by the companies listed below.
Automating lifts, tows, carts and AGVs
Human-operated tows, lifts, AGVs and other warehouse and factory vehicles has been a staple in material movement for decades. Now, with low-cost cameras, sensors and advanced vision and depth-sensing systems, they are slowly transitioning to more flexible mobile robots (AMRs) that can autonomously tow, lift and carry and can work in either autonomous or human-operated modes.
Of the top 20 industrial lift suppliers tabulated by Modern Materials Handling, only 5 offer kits or optional self-driving add-ons.
Vendors providing kits and systems for existing forklifts and carts to convert them to Vision Guided Vehicles (VGVs, AMRs) for line-side replenishment, pallet movement, etc. include:
- RoboCV is a Russian provider of autopilots for warehouse machines at Russian facilities for Samsung, VW and 3PLs. RoboCV also provides cloud-based task optimization and traffic control.
- Balyo, a French provider of autonomous vehicle kits to forklift OEMs Hyster and Yale.
- Seegrid, a Pittsburgh-based provider of vehicle autonomous kits for OEM Raymond, 3PLs and distribution centers of all types, also makes their own VGVs, and provides software and engineering systems to minimize human involvement and maximize VGV productivity.
Vendors providing AMRs, VGVs and AIVs (Autonomous Intelligent Vehicles) for goods-to-person, point-to-point, load transfer, restocking, etc. include:
- 6 River Systems
- Aethon
- Beijing Geekplus Technology (Geek+)
- Canvas Technology
- Clearpath’s OTTO robots
- Fetch Robotics
- Grenzebach
- Kuka
- Locus Robotics
- Mobile Industrial Robots
- Robotnik Automation
- Seegrid
- STILL
- Swisslog
- Toyota’s Autopilot
- Vecna Robotics
- and others
Grasping
Where humans surpass machines is in the quick visual determination of what to pick, how to grasp, and then move the item to wherever it needs to go. Until recently, this has been the missing link in automated fulfillment and one of the biggest challenges in robotics acceptance. A few vendors are perfecting the science that enables high speed random grasping from moving conveyors or bins:
- RightHand Robotics
- Universal Logic
- Kinema Systems
- Swisslog
- Soft Robotics
Vendors providing grasping capabilities in addition to autonomous mobility include:
- InVia Robotics
- IAM Robotics
- Magazino
- Dorabot
- GreyOrange (see below for details)
Indoor navigation
Navigation systems have changed along with all the other technological improvements and often don’t require floor grid markings, barcodes or extensive indoor localization and segregation systems such as those used by Kiva Systems (and subsequently Amazon). SLAM and combinations of floor grids, SLAM, path planning and mapping systems, indoor beacons, and collision avoidance systems are adding flexibility to swarms of point-to-point mobile robots and enabling traffic control and dynamic inventory placement.
Kiva look-alikes
In March 2012, in an effort to make their fulfillment centers as efficient as possible, Amazon acquired Kiva Systems for $775 million and almost immediately took them in-house, leaving a disgruntled set of Kiva customers who couldn’t expand and a larger group of prospective clients who were left with a technological gap and no solutions. I wrote about this gap and about the whole community of new providers that had sprung up to fill the void and were beginning to offer and demonstrate their solutions. Many of those new providers are listed above.
Recently, another set of competitors has emerged in this space. Chinese e-commerce giants Alibaba, JD (JingDong), VIPShop, Tencent and others have funded companies who copied the Kiva Systems formula to provide Kiva-like goods-to-person robot systems and dynamic free-form warehousing for their in-country fulfillment and distribution centers.
Now some of those companies are braving the prospect of IP infringement proceedings from Amazon and are expanding outside of China and SE Asia to Europe and America:
- Grey Orange Robotics has sites using their systems in Japan and Europe and exhibited at Europe’s Logimat trade show where they launched PickPal, an autonomous picking robot which can pick a wide variety of SKUs using machine vision and a scalable gripper system specifically suitable for high-volume order fulfillment.
- Beijing Geekplus (Geek+) Technology also has sites using their systems in Japan and Poland and had booths at MODEX and CeMAT trade shows to introduce Geek+ to the West.
- Xinyi Logistics Science & Technology (Alog) – has not yet ventured beyond China and SE Asia.
- Shanghai Express Warehouse (Quicktron / Flashhold) –
- Hanzhou Hikrobot Technology (HIK) – has not yet ventured beyond China and SE Asia.
Kiva alternatives
- Symbotic
- Swisslog
- Dematic
- Locus Robotics
- Fetch Robotics
[NOTE: The lists shown above are not fully comprehensive. The universe is much larger. I have some knowledge of the vendors shown and know that they are beyond pilot projects, researching and prototyping which was my criteria for including them.]