Engineers capture octopus arm’s intricate muscular architecture with an unprecedented computational model
A milestone in the study of octopus arms
Robot that watched surgery videos performs with skill of human doctor, researchers report
Watching surgery videos allows robot to perform with skill of human doctor
New TI MCUs enable edge AI and industry-leading real-time control to advance system efficiency, safety and sustainability
Close Enough for Rock ‘n Roll!
Too Many ‘Major AI Product Releases’ Not Ready for Prime Time
Back in my garage-band playing days, I remember turning to the group’s rhythm guitarist during a rehearsal and letting him know that his top strings were all flat.
To which he replied — with a toothy grin — “Close enough for Rock ‘n Roll!”
Unfortunately, that completely juvenile, “I”m-to-cool-to-give-a-damn?” swagger has been cropping-up all over the AI marketplace lately.
Last week, for example, ChatGPT-maker OpenAI released a new search engine to the world that some are heralding as a ‘Google-killer.’
But many people who actually used the search engine quickly discovered that the ‘fairy-dust-from-the-future’ was confidently bringing back text summaries of searches that were simply wrong.
Moreover, medical users of OpenAI’s Whisper transcription app are finding out that the tool — in some cases — is inserting ‘invented facts’ into the transcriptions.
Meaning that if a doctor has diagnosed someone with cancer, the resulting Whisper transcription may ‘invent’ a fact that contradicts the doctor’s diagnosis — or ‘invent’ a treatment that is not recommended for that form of cancer.
Oops.
Sadly, other — normally highly respected names in Big Tech — are also playing the same game.
Google’s recently popular NotebookLM, for example, has been hailed by some as ‘insanely magical’ for its ability to scrutinize a text document and then quickly auto-generate an audio discussion about that document by two, extremely human-sounding robotic voices.
The only problem: Turns-out, those cheery robotic voices also gleefully make-up facts not found in the source text.
And let’s not get started on Google Gemini’s initially bungled release of Gemini’s imaging capability back in February, which depicted America’s founding fathers — and Nazis — as racial minorities.
Meanwhile, even Apple is getting into the act.
In late October, the company breathlessly unveiled its supposedly ‘game-changing,’ much anticipated AI software update, dubbed ‘Apple Intelligence,’ which according to some, was destined to remake the world as we know it.
Instead, users quickly learned the ‘wunderkind’ AI writing and editing tools on board Apple Intelligence were actually much weaker versions of what you can get with the latest paid version of ChatGPT at 20-bucks-a-month.
Bottom-line: While many who follow tech closely are well aware of the Silicon Valley ethic, ‘Move Fast, Break Things and Apologize Afterwards’ we’ve reached a point where that bravado is endangering lives — and seriously eroding the public’s confidence in AI.
For example: Should we really be forced to put-up with a product used in a medical setting that could write down the wrong diagnosis and recommend the wrong treatment?
Should we really allow a product to stay on the market, even in experimental form, that auto-generates fictional interpretations of text documents — without an accompanying warning label?
Should we really be in awe of one of the top five most valuable companies on the planet, which pretends to release a ‘bleeding-edge,’ AI editing and writing tool — only to learn the app is actually generations behind the state-of-the-art?
No.
We shouldn’t.
Don’t get me wrong: I am in awe of many AI products that are truthfully marketed and advertised.
For example: I think OpenAI’s flagship product, ChatGPT, is an amazing tool for auto-writing and myriad other uses.
And I admire the fact that ChatGPT’s maker, OpenAI, has — from the very beginning — included a highly prominent warning label on the ChatGPT Web site that unequivocally declares the tool is prone to making-up facts.
But when the reverse is true, and we come across AI companies that are repeatedly releasing AI tools on the market that they fully realize are deeply flawed — and in some cases, even life-threatening — we have no choice but to brand them as who they really are:
Charlatans.
In other news and analysis on AI writing:
*The Waiting is the Hardest Part: No GPT-5 for 2024: Avid fans of ChatGPT — present company included — learned with some remorse that the tool will not be upgraded for a while.
That’s a blow to writers, given that the current version — ChatGPT-4 — seems to be best overall version of OpenAI’s software options for creative and nonfiction writing.
A major update would have most likely made it even better by far.
Still, we can hope for an update in 2025.
*Sweet Nothings: When ‘Whisper’ Medical Transcriptions Become Creative Writing: In a disturbing finding, many researchers are finding that Whisper — a transcription tool from ChatGPT-maker OpenAI — is making-up facts.
Observes lead writer Garance Burke: “Experts said that such fabrications are problematic because Whisper is being used in a slew of industries worldwide to translate and transcribe interviews, generate text in popular consumer technologies and create subtitles for videos.
“More concerning, they said, is a rush by medical centers to utilize Whisper-based tools to transcribe patients’ consultations with doctors.”
*Whisper Alternative Otter.ai Apparently Sticks to the Script: Writer Radhika Rajkumar advises that users of transcription tool Whisper — which has been found to make-up facts in the transcriptions it renders — should use Otter.ai instead.
Observes Rajkumar: “While you’re waiting for OpenAI to resolve the issue, we recommend trying Otter.ai, a journalist-trusted AI transcription tool.”
*Notion: Promising an AI Email Inbox That Thinks Like You: Notion is promising to deliver a new AI-powered app in early 2025 that will highly automate and customize every facet of your email experience.
Observes writer Emma Roth: “Much like Notion’s other tools, the company says Mail will distill email down to its building blocks, allowing you to create an inbox with views, layouts and actions tailored to your preferences.
“You can also use Notion AI to automatically organize, archive, or draft emails based on a prompt.”
*Google’s Gemini Comes to Gmail-on-the-Web: Leaving no stone unturned, Google has decided to offer AI help when you’re writing emails with Gmail on the Web.
Observes writer Emma Roth: “In addition to generating an email draft, ‘Help me write’ can also provide suggestions on how to formalize, elaborate, or shorten a message.
“Google’s ‘Help me write’ feature is only available to users who subscribe to Google One AI Premium or have the Gemini add-on for Workspace.”
*Microsoft Notepad Gets the AI Treatment: Maybe Even Your Grocery List Will Read Like Poetry: Like many other tech titans, Microsoft continues to make good on its intention to embed AI everywhere.
This time, AI is coming to its Notepad app.
Dubbed ‘Rewrite,’ the new feature “promises to spruce-up your text with the help of AI.
“Using an AI model called GPT, Rewrite can revise sentences, modify the tone, or alter the length of your text,” according to writer Lance Whitney.
*Claude Comes to Your Desktop: Because Browser AI is So 2024: Users of Claude — a top alternative to ChatGPT — can now work with the ‘auto-writer and more’ directly from Windows and Mac desktops.
Observes writer Lance Whitney: “The new apps work similarly to the Web site and are available for free users and paid subscribers.
“For now, the apps are tagged with a beta label, which may indicate that Anthropic is still tweaking them.”
*Living the AI Dream: Reducing Email Reading Time By 97%: Users of AI-powered data-analysis tool Snowflake report that the platform is saving companies significant time by auto-reading emails.
Case in point: Thomas Bodenski, CEO, TS Imagine, who reports that he’s using Snowflake’s AI to scan incoming emails for ‘crucial, actionable events.’
The result: Bodenski has reduced the time needed to process, understand and act on those emails by 97%.
*AI Big Picture: AI Now ‘Pitch Perfect’ for Most Marketers: A new study from The University of Pennsylvania finds that 62% of workers in marketing and sales are now using AI as a core tool.
Observes Stefano Puntoni, a marketing professor at the university: “Generative AI has rapidly evolved from a tool of experimentation to a core driver of business transformation.
“Companies are no longer just exploring AI’s potential.
“They are embedding it into their strategies to scale growth, streamline operations and enhance decision-making.”

Share a Link: Please consider sharing a link to https://RobotWritersAI.com from your blog, social media post, publication or emails. More links leading to RobotWritersAI.com helps everyone interested in AI-generated writing.
–Joe Dysart is editor of RobotWritersAI.com and a tech journalist with 20+ years experience. His work has appeared in 150+ publications, including The New York Times and the Financial Times of London.
The post Close Enough for Rock ‘n Roll! appeared first on Robot Writers AI.
Washbasin-cleaning robot can imitate human motions and adapt its knowledge flexibly to different situations
Artificial magnetic muscles can support tensile stresses up to 1,000 times their own weight
NVIDIA Advances Robot Learning and Humanoid Development With New AI and Simulation Tools
Robot Talk Episode 97 – Pratap Tokekar
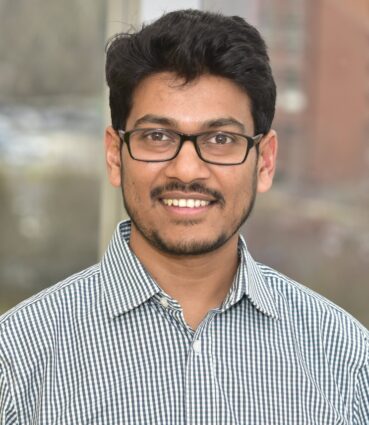
Claire chatted to Pratap Tokekar from the University of Maryland about how teams of robots with different capabilities can work together.
Pratap Tokekar is an Associate Professor in the Department of Computer Science and the Institute for Advanced Computer Studies at the University of Maryland, and an Amazon Scholar. Previously, he was a Postdoctoral Researcher at the GRASP lab of University of Pennsylvania and later, an Assistant Professor at Virginia Tech. He has a degree in Electronics and Telecommunication from the College of Engineering Pune in India and a Ph.D. in Computer Science from the University of Minnesota. He received the Amazon Research Award in 2022, and the NSF CAREER award in 2020.