Machine Automation Taken to the Next Level
How Are Robots Tested for Harsh Conditions?
Packaging the Future
Wireless Charging to Bring ‘True Autonomy’ to Mobility Era in Robotics
Robotics Industry Set for Seismic Change as Growth Shifts from Fixed Automation to Mobile Systems in Enterprise
#299: On the Novelty Effect in Human-Robot Interaction, with Catharina Vesterager Smedegaard
In this episode, we take a closer look at the effect of novelty in human-robot interaction. Novelty is the quality of being new or unusual.
The typical view is that while something is new, or “a novelty”, it will initially make us behave differently than we would normally. But over time, as the novelty wears off, we will likely return to our regular behaviors. For example, a new robot may cause a person to behave differently initially, as its introduced into the person’s life, but after some time, the robot won’t be as exciting, novel and motivating, and the person might return to their previous behavioral patterns, interacting less with the robot.
To find out more about the concept of novelty in human-robot interactions, our interviewer Audrow caught up with Catharina Vesterager Smedegaard, a PhD-student at Aarhus University in Denmark, whose field of study is Philosophy.
Catharina sees novelty differently to how we typically see it. She thinks of it as projecting what we don’t know onto what we already know, which has implications for how human-robot interactions are designed and researched. She also speaks about her experience in philosophy more generally, and gives us advice on philosophical thinking.
Catharina Vesterager Smedegaard
Catharina Vesterager Smedegaard started as a PhD-student the 1st of December 2017. She has a BA and MA in philosophy. In autumn 2015, Catharina interned at the research group PENSOR (the present RUR), where she first became interested in Social Robotics and formed the idea for her MA thesis.
Links
3 HR Headaches Robotics Can Solve
ElectroCraft Product for Robotics Technologies Across Markets
Call for robot holiday videos 2019
That’s right! You better not run, you better not hide, you better watch out for brand new robot holiday videos on Robohub!
Drop your submissions down our chimney at sabine.hauert@robohub.org and share the spirit of the season.
For inspiration, check out submissions from last year.
Data-driven deep reinforcement learning
By Aviral Kumar
One of the primary factors behind the success of machine learning approaches in open world settings, such as image recognition and natural language processing, has been the ability of high-capacity deep neural network function approximators to learn generalizable models from large amounts of data. Deep reinforcement learning methods, however, require active online data collection, where the model actively interacts with its environment. This makes such methods hard to scale to complex real-world problems, where active data collection means that large datasets of experience must be collected for every experiment – this can be expensive and, for systems such as autonomous vehicles or robots, potentially unsafe. In a number of domains of practical interest, such as autonomous driving, robotics, and games, there exist plentiful amounts of previously collected interaction data which, consists of informative behaviours that are a rich source of prior information. Deep RL algorithms that can utilize such prior datasets will not only scale to real-world problems, but will also lead to solutions that generalize substantially better. A data-driven paradigm for reinforcement learning will enable us to pre-train and deploy agents capable of sample-efficient learning in the real-world.
In this work, we ask the following question: Can deep RL algorithms effectively leverage prior collected offline data and learn without interaction with the environment? We refer to this problem statement as fully off-policy RL, previously also called batch RL in literature. A class of deep RL algorithms, known as off-policy RL algorithms can, in principle, learn from previously collected data. Recent off-policy RL algorithms such as Soft Actor-Critic (SAC), QT-Opt, and Rainbow, have demonstrated sample-efficient performance in a number of challenging domains such as robotic manipulation and atari games. However, all of these methods still require online data collection, and their ability to learn from fully off-policy data is limited in practice. In this work, we show why existing deep RL algorithms can fail in the fully off-policy setting. We then propose effective solutions to mitigate these issues.
ROBOTT-NET pilot project: Cobots for safe and cheap assembly of frequency converters
At Danfoss in Gråsten, the Danish Technological Institute (DTI) is testing, as part of a pilot project in the European robot network ROBOTT-NET, several robot technologies: Manipulation using force sensors, simpler separation of items and a 3D-printed three-in-one gripper for handling capacitors, nuts and a socket handle.
“The set-updemonstrates various techniques that provide a cheaper solution, increased robustness and increased safety for operators”, says Søren Peter Johansen Technology Manager at DTI.
“For example, there is a force-torque sensor in the robot which is used to manoeuvre things carefully into place, and also to increase the confidence of the operators. The force-torque sensor will sense and prevent collisions with people before the built-in safety features of the robot stop the robot arm. Thus, we can also increase safety and confidence by working with collaborative robots”, he says.
Increased effectiveness
Danfoss in Gråsten has tested the robot in connection with the company’s production of frequency converters.
“A frequency converter contains, amongst other things, capacitors assembled in groups of two or four. We have automated this process in that a robot picks up a capacitor and puts it into a fixture. For the capacitor to be correctly placed in the fixture, the electrodes must point in the right direction. Therefore, a camera sees how the electrodes are oriented in the gripper, and orients correctly before it is placed in the fixture”, explains Søren Peter Johansen.
“Then we pick a nut that has to be put on and the robot picks up the socket handle and screws the nut tight. And meanwhile the operator can do something else and thus increases efficiency”, he elaborates.
Three reasons to automate
Peter Lund Andersen, Senior Manufacturing Specialist at Danfoss, says that Danfoss is automating for several reasons:
“It is primarily about work and environmental considerations – better ergonomics and less heavy lifting. We believe that with robots, that can do the work uniformly each time, we can increase the quality of our products while maintaining safety and reducing costs”, he says, adding “The cooperation with DTI gives us the opportunity to come out and try some new things. Some things that aren’t quite mature yet and can’t just be taken off a shelf. That way we are at the forefront with everything.”
If you want to watch more videos, you can explore ROBOTT-NET’s pilot projects on our YouTube-channel.
Helping machines perceive some laws of physics
Image: Christine Daniloff, MIT
Humans have an early understanding of the laws of physical reality. Infants, for instance, hold expectations for how objects should move and interact with each other, and will show surprise when they do something unexpected, such as disappearing in a sleight-of-hand magic trick.
Now MIT researchers have designed a model that demonstrates an understanding of some basic “intuitive physics” about how objects should behave. The model could be used to help build smarter artificial intelligence and, in turn, provide information to help scientists understand infant cognition.
The model, called ADEPT, observes objects moving around a scene and makes predictions about how the objects should behave, based on their underlying physics. While tracking the objects, the model outputs a signal at each video frame that correlates to a level of “surprise” — the bigger the signal, the greater the surprise. If an object ever dramatically mismatches the model’s predictions — by, say, vanishing or teleporting across a scene — its surprise levels will spike.
In response to videos showing objects moving in physically plausible and implausible ways, the model registered levels of surprise that matched levels reported by humans who had watched the same videos.
“By the time infants are 3 months old, they have some notion that objects don’t wink in and out of existence, and can’t move through each other or teleport,” says first author Kevin A. Smith, a research scientist in the Department of Brain and Cognitive Sciences (BCS) and a member of the Center for Brains, Minds, and Machines (CBMM). “We wanted to capture and formalize that knowledge to build infant cognition into artificial-intelligence agents. We’re now getting near human-like in the way models can pick apart basic implausible or plausible scenes.”
Joining Smith on the paper are co-first authors Lingjie Mei, an undergraduate in the Department of Electrical Engineering and Computer Science, and BCS research scientist Shunyu Yao; Jiajun Wu PhD ’19; CBMM investigator Elizabeth Spelke; Joshua B. Tenenbaum, a professor of computational cognitive science, and researcher in CBMM, BCS, and the Computer Science and Artificial Intelligence Laboratory (CSAIL); and CBMM investigator Tomer D. Ullman PhD ’15.
Mismatched realities
ADEPT relies on two modules: an “inverse graphics” module that captures object representations from raw images, and a “physics engine” that predicts the objects’ future representations from a distribution of possibilities.
Inverse graphics basically extracts information of objects — such as shape, pose, and velocity — from pixel inputs. This module captures frames of video as images and uses inverse graphics to extract this information from objects in the scene. But it doesn’t get bogged down in the details. ADEPT requires only some approximate geometry of each shape to function. In part, this helps the model generalize predictions to new objects, not just those it’s trained on.
“It doesn’t matter if an object is rectangle or circle, or if it’s a truck or a duck. ADEPT just sees there’s an object with some position, moving in a certain way, to make predictions,” Smith says. “Similarly, young infants also don’t seem to care much about some properties like shape when making physical predictions.”
These coarse object descriptions are fed into a physics engine — software that simulates behavior of physical systems, such as rigid or fluidic bodies, and is commonly used for films, video games, and computer graphics. The researchers’ physics engine “pushes the objects forward in time,” Ullman says. This creates a range of predictions, or a “belief distribution,” for what will happen to those objects in the next frame.
Next, the model observes the actual next frame. Once again, it captures the object representations, which it then aligns to one of the predicted object representations from its belief distribution. If the object obeyed the laws of physics, there won’t be much mismatch between the two representations. On the other hand, if the object did something implausible — say, it vanished from behind a wall — there will be a major mismatch.
ADEPT then resamples from its belief distribution and notes a very low probability that the object had simply vanished. If there’s a low enough probability, the model registers great “surprise” as a signal spike. Basically, surprise is inversely proportional to the probability of an event occurring. If the probability is very low, the signal spike is very high.
“If an object goes behind a wall, your physics engine maintains a belief that the object is still behind the wall. If the wall goes down, and nothing is there, there’s a mismatch,” Ullman says. “Then, the model says, ‘There’s an object in my prediction, but I see nothing. The only explanation is that it disappeared, so that’s surprising.’”
Violation of expectations
In development psychology, researchers run “violation of expectations” tests in which infants are shown pairs of videos. One video shows a plausible event, with objects adhering to their expected notions of how the world works. The other video is the same in every way, except objects behave in a way that violates expectations in some way. Researchers will often use these tests to measure how long the infant looks at a scene after an implausible action has occurred. The longer they stare, researchers hypothesize, the more they may be surprised or interested in what just happened.
For their experiments, the researchers created several scenarios based on classical developmental research to examine the model’s core object knowledge. They employed 60 adults to watch 64 videos of known physically plausible and physically implausible scenarios. Objects, for instance, will move behind a wall and, when the wall drops, they’ll still be there or they’ll be gone. The participants rated their surprise at various moments on an increasing scale of 0 to 100. Then, the researchers showed the same videos to the model. Specifically, the scenarios examined the model’s ability to capture notions of permanence (objects do not appear or disappear for no reason), continuity (objects move along connected trajectories), and solidity (objects cannot move through one another).
ADEPT matched humans particularly well on videos where objects moved behind walls and disappeared when the wall was removed. Interestingly, the model also matched surprise levels on videos that humans weren’t surprised by but maybe should have been. For example, in a video where an object moving at a certain speed disappears behind a wall and immediately comes out the other side, the object might have sped up dramatically when it went behind the wall or it might have teleported to the other side. In general, humans and ADEPT were both less certain about whether that event was or wasn’t surprising. The researchers also found traditional neural networks that learn physics from observations — but don’t explicitly represent objects — are far less accurate at differentiating surprising from unsurprising scenes, and their picks for surprising scenes don’t often align with humans.
Next, the researchers plan to delve further into how infants observe and learn about the world, with aims of incorporating any new findings into their model. Studies, for example, show that infants up until a certain age actually aren’t very surprised when objects completely change in some ways — such as if a truck disappears behind a wall, but reemerges as a duck.
“We want to see what else needs to be built in to understand the world more like infants, and formalize what we know about psychology to build better AI agents,” Smith says.
RoboNet: A dataset for large-scale multi-robot learning
By Sudeep Dasari
This post is cross-listed at the SAIL Blog and the CMU ML blog.
In the last decade, we’ve seen learning-based systems provide transformative solutions for a wide range of perception and reasoning problems, from recognizing objects in images to recognizing and translating human speech. Recent progress in deep reinforcement learning (i.e. integrating deep neural networks into reinforcement learning systems) suggests that the same kind of success could be realized in automated decision making domains. If fruitful, this line of work could allow learning-based systems to tackle active control tasks, such as robotics and autonomous driving, alongside the passive perception tasks to which they have already been successfully applied.
While deep reinforcement learning methods – like Soft Actor Critic – can learn impressive motor skills, they are challenging to train on large and broad data that is not from the target environment. In contrast, the success of deep networks in fields like computer vision was arguably predicated just as much on large datasets, such as ImageNet, as it was on large neural network architectures. This suggests that applying data-driven methods to robotics will require not just the development of strong reinforcement learning methods, but also access to large and diverse datasets for robotics. Not only can large datasets enable models that generalize effectively, but they can also be used to pre-train models that can then be adapted to more specialized tasks using much more modest datasets. Indeed, “ImageNet pre-training” has become a default approach for tackling diverse tasks with small or medium datasets – like 3D building reconstruction. Can the same kind of approach be adopted to enable broad generalization and transfer in active control domains, such as robotics?
Unfortunately, the design and adoption of large datasets in reinforcement learning and robotics has proven challenging. Since every robotics lab has their own hardware and experimental set-up, it is not apparent how to move towards an “ImageNet-scale” dataset for robotics that is useful for the entire research community. Hence, we propose to collect data across multiple different settings, including from varying camera viewpoints, varying environments, and even varying robot platforms. Motivated by the success of large-scale data-driven learning, we created RoboNet, an extensible and diverse dataset of robot interaction collected across four different research labs. The collaborative nature of this work allows us to easily capture diverse data in various lab settings across a wide variety of objects, robotic hardware, and camera viewpoints. Finally, we find that pre-training on RoboNet offers substantial performance gains compared to training from scratch in entirely new environments.
Our goal is to pre-train reinforcement learning models on a sufficiently diverse dataset and then transfer knowledge (either zero-shot or with fine-tuning) to a different test environment.
Collecting RoboNet
RoboNet consists of 15 million video frames, collected by different robots interacting with different objects in a table-top setting. Every frame includes the image recorded by the robot’s camera, arm pose, force sensor readings, and gripper state. The collection environment, including the camera view, the appearance of the table or bin, and the objects in front of the robot are varied between trials. Since collection is entirely autonomous, large amounts can be cheaply collected across multiple institutions. A sample of RoboNet along with data statistics is shown below:
A sample of data from RoboNet alongside a summary of the current dataset. Note that any GIF compression artifacts in this animation are not present in the dataset itself.
How can we use RoboNet?
After collecting a diverse dataset, we experimentally investigate how it can be used to enable general skill learning that transfers to new environments. First, we pre-train visual dynamics models on a subset of data from RoboNet, and then fine-tune them to work in an unseen test environment using a small amount of new data. The constructed test environments (one of which is visualized below) all include different lab settings, new cameras and viewpoints, held-out robots, and novel objects purchased after data collection concluded.
Example test environment constructed in a new lab, with a temporary uncalibrated camera, and a new Baxter robot. Note that while Baxters are present in RoboNet that data is not included during model pre-training.
After tuning, we deploy the learned dynamics models in the test environment to perform control tasks – like picking and placing objects – using the visual foresight model based reinforcement learning algorithm. Below are example control tasks executed in various test environments.
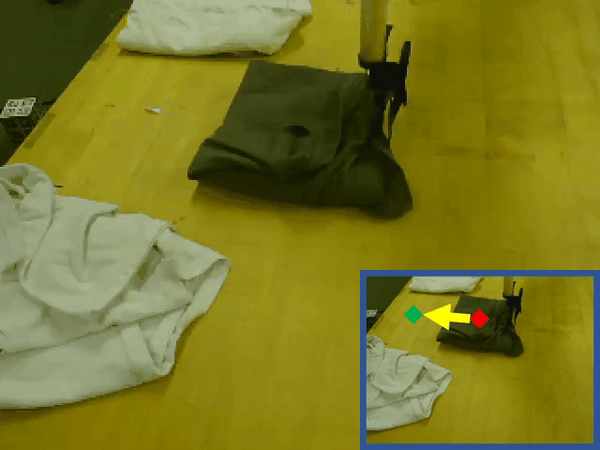
Kuka can align shirts next to the others
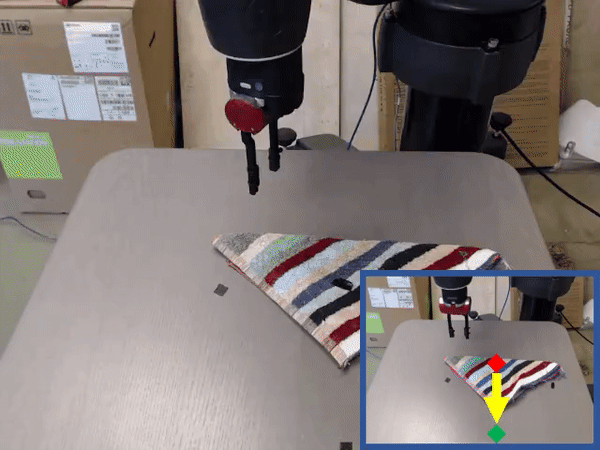
Baxter can sweep the table with cloth
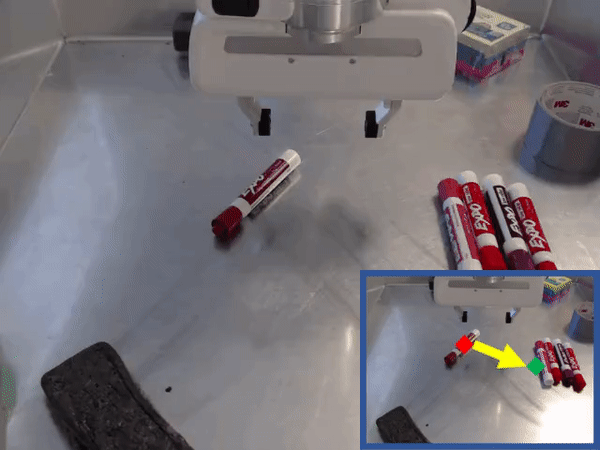
Franka can grasp and reposition the markers
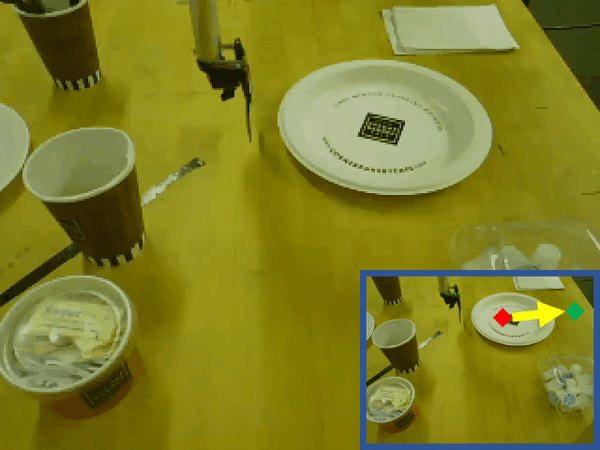
Kuka can move the plate to the edge of the table
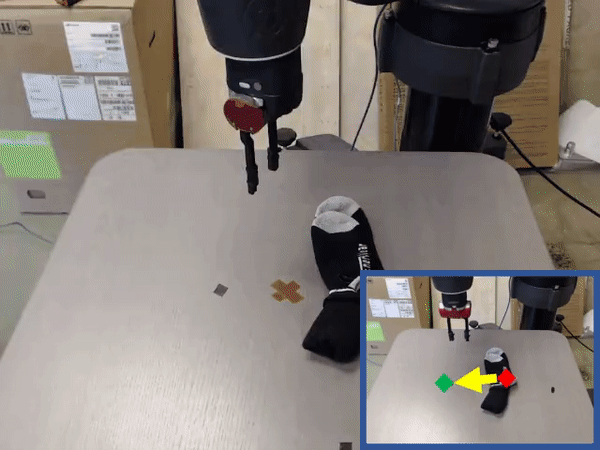
Baxter can pick up and reposition socks
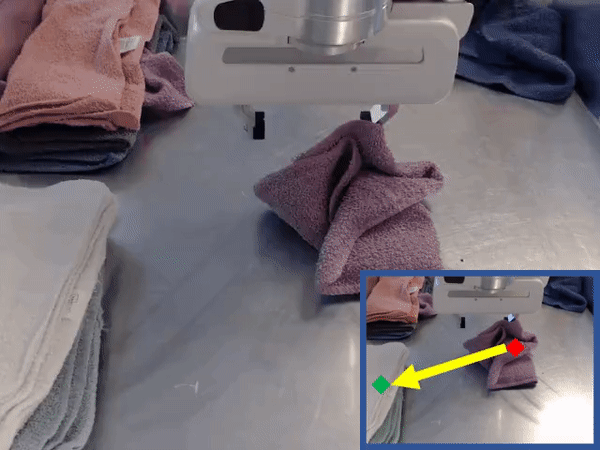
Franka can stack the towel on the pile
Here you can see examples of visual foresight fine-tuned to perform basic control tasks in three entirely different environments. For the experiments, the target robot and environment was subtracted from RoboNet during pre-training. Fine-tuning was accomplished with data collected in one afternoon.
We can now numerically evaluate if our pre-train controllers can pick up skills in new environments faster than a randomly initialized one. In each environment, we use a standard set of benchmark tasks to compare the performance of our pre-trained controller against the performance of a model trained only on data from the new environment. The results show that the fine-tuned model is ~4x more likely to complete the benchmark task than the one trained without RoboNet. Impressively, the pre-trained models can even slightly outperform models trained from scratch on significantly (5-20x) more data from the test environment. This suggests that transfer from RoboNet does indeed offer large performance gains compared to training from scratch!
We compare the performance of fine-tuned models against their counterparts trained from scratch in two different test environments (with different robot platforms).
Clearly fine-tuning is better than training from scratch, but is training on all of RoboNet always the best way to go? To test this, we compare pre-training on various subsets of RoboNet versus training from scratch. As seen before, the model pre-trained on all of RoboNet (excluding the Baxter platform) performs substantially better than the random initialization model. However, the “RoboNet pre-trained” model is outperformed by a model trained on a subset of RoboNet data collected on the Sawyer robot – the single-arm variant of Baxter.
Models pre-trained on various subsets of RoboNet are compared to one trained from scratch in an unseen (during pre-training) Baxter control environment
The similarities between the Baxter and Sawyer likely partly explain our results, but why does simply adding data to the training set hurt performance after fine-tuning? We theorize that this effect occurs due to model under-fitting. In other words, RoboNet is an extremely challenging dataset for a visual dynamics model, and imperfections in the model predictions result in bad control performance. However, larger models with more parameters tend to be more powerful, and thus make better predictions on RoboNet (visualized below). Note that increasing the number of parameters greatly improves prediction quality, but even large models with 500M parameters (middle column in the videos below) are still quite blurry. This suggests ample room for improvement, and we hope that the development of newer more powerful models will translate to better control performance in the future.
We compare video prediction models of various size trained on RoboNet. A 75M parameter model (right-most column) generates significantly blurrier predictions than a large model with 500M parameters (center column).
Final Thoughts
This work takes the first step towards creating learned robotic agents that can operate in a wide range of environments and across different hardware. While our experiments primarily explore model-based reinforcement learning, we hope that RoboNet will inspire the broader robotics and reinforcement learning communities to investigate how to scale model-based or model-free RL algorithms to meet the complexity and diversity of the real world.
Since the dataset is extensible, we encourage other researchers to contribute the data generated from their experiments back into RoboNet. After all, any data containing robot telemetry and video could be useful to someone else, so long as it contains the right documentation. In the long term, we believe this process will iteratively strengthen the dataset, and thus allow our algorithms that use it to achieve greater levels of generalization across tasks, environments, robots, and experimental set-ups.
For more information please refer to the the project website. We’ve also open sourced our code-base and the entire RoboNet dataset.
Finally, I would like to thank Sergey Levine, Chelsea Finn, and Frederik Ebert for their helpful feedback on this post.
This article was initially published on the BAIR blog, and appears here with the authors’ permission.
This blog post was based on the following paper:
- RoboNet: Large-Scale Multi-Robot Learning.
S. Dasari, F. Ebert, S. Tian, S. Nair, B. Bucher, K. Schmeckpeper, S. Singh, S. Levine, C. Finn.
In Conference on Robot Learning, 2019.
The 5G report card: Building today’s smart IoT ecosystem
The elephant in the room loomed large two weeks ago at the inaugural Internet of Things Consortium (IoTC) Summit in New York City. Almost every presentation began apologetically with the refrain, “In a 5G world” practically challenging the industry’s rollout goals. At one point Brigitte Daniel-Corbin, IoT Strategist with Wilco Electronic Systems, sensed the need to reassure the audience by exclaiming, ‘its not a matter of if, but when 5G will happen!’ Frontier Tech pundits too often prematurely predict hyperbolic adoption cycles, falling into the trap of most soothsaying visions. The IoTC Summit’s ability to pull back the curtain left its audience empowered with a sober roadmap forward that will ultimately drive greater innovation and profit.
The industry frustration is understandable as China announced earlier this month that 5G is now commercially available in 50 cities, including: Beijing, Shanghai and Shenzhen. In fact, the communist state beat its own 2020 objectives by rolling out the technology months ahead of plan. Already more than 10 million cellular customers have signed up for the service. China has made upgrading its cellular communications a national priority with more than 86,000 5G base stations installed to date and another 130,000 5G base stations to go live by the end of the year. In the words of Wang Xiaochu, president of China Unicom, “The commercialization of 5G technology is a great measure of [President] Xi Jinping’s strategic aim of turning China into a cyber power, as well as an important milestone in China’s information communication industry development.” By contrast the United States is still testing the technology in a number of urban zones. If a recent PC Magazine review of Verizon’s Chicago pilot is any indication of the state of the technology, the United States is very far from catching up. As one reporter complains, “I walked around for three hours and found that coverage is very spotty.”
Last year, President Trump donning a hardhat declared “My administration is focused on freeing up as much wireless spectrum as needed [to make 5G possible].” The importance of Trump’s promotional event in April could not be more understated, as so much of the future of autonomous driving, additive manufacturing, collaborative robotics, shipping & logistics, smart city infrastructure, Internet of Things (IoT), and virtual & augmented reality relies on greater bandwidth. Most experts predict that 5G will offer a 10 to 100 times improvement over fourth generation wireless. Els Baert of NetComm explains, “The main advantage that 5G offers over 4G LTE is faster speeds — primarily because there will be more spectrum available for 5G, and it uses more advanced radio technology. It will also deliver much lower latency than 4G, which will enable new applications in the [Internet of Things] space.” Unfortunately, since Trump’s photo op, the relationship with China has worsened so much that US carriers are now blocked from doing business with the largest supplier of 5G equipment, Huawei. This leaves the United States with only a handful of suppliers, including market leaders Nokia and Ericsson. The limited supply chain is exasperated by how little America is spending on upgrading its telecommunications, according to Deloitte “we conclude that the United States underspent China in wireless infrastructure by $8 billion to $10 billion per year since 2015.”
The current state of the technology (roadblocks and all) demands fostering an innovation ecosystem today that parallels the explosion of new services for the 5G economy. As McKinsey reports there are more than 25 billion connected IoT devices currently, which is estimated to grow to more than 75 billion by 2025 with the advent of fifth generation wireless. The study further cites, “General Electric projects that IoT will add $10 to $15 trillion to a worldwide Global Domestic Product (GDP) growth by 2030. To put that into perspective, that number is equivalent to China’s entire current economy.” Regrettably, most of the available 5G accelerators in the USA are built to showcase virtual and augmented reality instead of fostering applications for the larger opportunity of business-to-business services. According to Business Insider “IoT solutions will reach $6 trillion by 2021,” across a wide spectrum of industries, including: healthcare, manufacturing, logistics, energy, smart homes, transportation and urban development. In fact, hardware will only account for about one-third of the new revenues (and VR/AR headsets comprise considerably less).
It is challenging for publicly traded companies (like T-Mobile, Verizon & AT&T), whose stock performance is so linked to the future of next generation wireless. Clearly, market makers are overly excited by the unicorns of Oculus (acquired by Facebook for $2 billion in 2014) and Magic Leap (valued at $4.5 billion in 2016) more than IoT sensors for robotic recycling, agricultural drones, and fuel efficient rectors. However, based upon the available data, the killer app for 5G will be found in industry not digital theatrics. This focus on theatrics is illustrated in one of the few statements online by Verizon’s Christian Guirnalda, Director of its 5G Labs, boasting “We’re literally making holograms here using a dozen of different cameras in a volumetric capture studio to create near real-time images of what people and products look like in 3D.” A few miles north of Verizon 5G Labs, New York City’s hospitals are overcrowded with patients and data leading to physical and virtual latency issues. Verizon could enable New York’s hospitals with faster network speeds to treat more patients in economically challenged neighborhoods remotely. Already, 5G threatens to exasperate the digital divide in the United States by targeting affluent communities for its initial rollout. By investing in more high-speed telemedicine applications, the telecommunications giant could potentially enable less privileged patients access to better care, which validates the need for increased government spending. Guirnalda’s Lab would be better served by applying the promise of 5G to solve these real-life urban challenges from mass transit to food scarcity to access to healthcare.
The drawback with most corporate 5G incubators is their windows are opaque – forcing inventors to experiment inside, while the real laboratory is bustling outside. The United Nations estimates by 2050 seventy percent of the world’s population will be urban. While most of this growth will take place in developing countries (i.e., Africa and Asia) already 80% of global GDP is generated in cities. The greatest challenge for the 21st century will be managing the sustainable development of these populations. At last month’s UN “World Cities Day,” the diplomatic body stated that 5G “big data technologies and cloud-computing offer the potential to enhance urban operations, functions, services, designs, strategies and policies.” The UN’s statement did not fall on deaf ears, even President Trump strained to comfort his constituents last month with the confession, “I asked Tim Cook to see if he could get Apple involved in building 5G in the U.S. They have it all – Money, Technology, Vision & Cook!”
Going to CES? Join me for my panel on Retail Robotics January 8th at 10am, Las Vegas Convention Center.